With psychiatric disorders rising by the year, there is an urgent need for measures to address them as a social issue, as they also have a substantial economic impact. Unlike blood glucose levels, blood pressure, and other useful biological markers, there are no objective measurements of such disorders, so no effective and revolutionary cures have yet been established. However, it would be fair to say that therapies have entered a new stage with the widespread use of MRI and the emergence of a more advanced technique called fMRI (Functional MRI), which enables brain circuits and their activity to be visualized in real time.
Special Feature 1 – Brain, Body, and Mind Visualizing brain activity with fMRI
composition by Takakazu Kawasaki
Having already been on the rise for some years, psychiatric disorders such as depression and schizophrenia have surged during the COVID-19 pandemic.
Looking at the conditions for which patients in Japan are treated, psychiatric disorders account for approximately 4.19 million patients in 2017, followed by diabetes at around 3.29 million (both figures from the Ministry of Health, Labour and Welfare’s 2017 Patient Survey). A number of conditions fall into the category of psychiatric disorders, including mood and affective disorders such as depression and bipolar affective disorder (bipolar disorder); schizophrenia, schizotypal and delusional disorders; and neurotic, stress-related and somatoform disorders such as panic disorder and post-traumatic stress disorder (PTSD). In the Sixth Medical Care Plan (2013), psychiatric disorders were added to the existing list of four major diseases (cancer, heart attack, stroke, and diabetes), becoming the fifth major disease to be the subject of focused measures by the health ministry. This indicates the scale of the increase in the number of patients with psychiatric disorders over the last 10 to 20 years.
In the U.S., the prevalence of depression has risen sharply by about 1.7 times in just a few years, from 8.7% in 2017–2018 to 14.4% in April 2020, when COVID-19 was just starting to spread across the globe. Estimates suggest that its prevalence is likely to have more than doubled by now. Japan can be expected to have seen a similar surge in psychiatric disorders to that in the U.S.
Younger women are more prone to PTSD
I conduct collaborative research with a major telecommunications operator KDDI, and we have found that addictions to games, smartphones, and the internet have all increased since the pandemic began. The risk of dependence on such IT devices tends to be several times higher in younger people. Suicides among young women have been on the rise since August 2020 or thereabouts. From an online study of the extent of the risk of developing a psychiatric disorder conducted among several thousand people, we discovered that young women have a strong tendency to develop PTSD.
Psychiatric disorders are not only a problem for the sufferers themselves. They also place a substantial burden on patients’ families, as sufferers need people to support and assist them. The social cost of depression alone in Japan is said to be about “2 trillion. Schizophrenia involves a lengthy period of hospitalization and therefore costs society around “3 trillion. When developmental disorders and the like are included, the total cost to society of psychiatric disorders could well be in the region of “10 trillion.
According to the World Health Organization (WHO), depression was at the third place among leading causes of burden of disease (DALYs) in 2004, expected in the first place by 2030. Psychiatric disorders are becoming a global problem.
- * The loss caused to society by disease. Calculated using the formula Years Lived with Disability + Years of Life Lost.
Blood tests are available for other diseases, such as diabetes. If someone is suspected of having heart disease, an electrocardiogram is used to test their heart. Chest X-rays are taken to confirm lung cancer or pneumonia.
However, there are no biological markers (measurement data) for psychiatric disorders, despite their immense social impact. Tests based on interviewing the patient are carried out to diagnose whether or not they have depression.
The patient health questionnaire takes the form of nine questions on topics ranging from (1) depressed mood, (2) loss of interest/pleasure in doing things, and (3) weight/appetite loss/gain, to (9) suicidal thoughts/attempts. If six or more of them are applicable to the patient, they are diagnosed with depression. The patient will often have their initial medical examination with a general physician, but even when a patient is seen by specialist physicians in psychiatric or psychosomatic medicine, the conformity in diagnosis (kappa coefficient) between multiple specialists is just 28%. Physicians’ diagnoses do not agree in almost three-quarters of cases.
A technology for visualizing hemodynamic reactions
Before getting onto the main subject of this article, I will just outline why I ended up researching psychiatric disorders even though I majored in physics.
When I was an undergraduate in the Faculty of Science, I wanted to pursue further studies in physics. Among the various options available to me were nuclear fusion, biophysics and neuroscience. While I was fretting about which path of study to choose, I realized that it was my brain puzzling over this decision, and I thought it might be interesting to use the techniques of physics to shed light on the mechanisms of the troubled brain. At graduate school, I undertook neuroscience research with the aid of techniques from theoretical physics, which involve using mathematics. One tool I found tremendously helpful in my research was a technology for visualizing hemodynamic reactions using functional magnetic resonance imaging (fMRI), the principles of which were discovered in the early 1990s by Professor Seiji Ogawa (Special University Professor Emeritus, Tohoku Fukushi University).
When the neurons in the brain are active, they consume more sugar as energy than when they are at rest, which means they require oxygen. Oxygen is carried by hemoglobin in the blood, and oxyhemoglobin (hemoglobin to which oxygen is attached) has a different magnetic susceptibility (the measurement of how easily it can be magnetized) from deoxyhemoglobin, whose oxygen has been removed.
An fMRI device emits radio waves (high-frequency electromagnetic waves) to cause magnetic resonance and the difference in magnetic susceptibility makes it possible to indirectly estimate brain activity from the quantity of oxygen, allowing us to determine that there is a lot of oxygen in a particular part of the brain or that oxygen levels in another are low. fMRI uses the same machine as medical MRI, but switching the sequence (program) enables us to investigate brain circuits.
While the brain accounts for only around 3% of our total body mass, its energy consumption accounts for about 20% of all energy consumed by the body. What is more, there is little difference in the amount of oxygen consumed by the brain between times when we feel that we are thinking really hard and times when, although not asleep, we are merely staring aimlessly into space. Consumption when we are not thinking of anything in particular is about 95% of the amount consumed when our brains are working flat out.
Incidentally, brain activity can also be measured by means of techniques such positron emission tomography (PET), which uses radioactive material, but fMRI is currently the best method of measuring brain activity. It can display images more or less in real time, taking just 10 minutes to measure a patient.
Diagnosis of psychiatric disorders by type
When fMRI is used to examine changes in various regions of the brain over time, we can calculate the degree of correlation between one region and another. If the change in activity is similar, it is described as having a positive correlation (>0), while if it is the complete opposite, it has a negative correlation (<0). If activity in the two regions is unrelated, it is described as having a zero correlation.
To look at this in more specific terms, let us say the brain is divided into 300 small regions, for example, and calculate the number of correlations between them. If we divide the brain into 300 small regions, the number of combinations of correlations is 300×299÷2, which is approximately 45,000 combinations. We divide the number by 2 because, when thinking about combinations, the correlation between region A and region B is the same as the correlation between region B and region A.
When we investigate the correlations between different regions, we obtain data showing regions with a positive correlation, regions with a negative correlation, and regions with zero correlation. We call this information about the brain’s circuits “brain functional connectivity networks.”
Furthermore, when we use artificial intelligence (AI) to analyze the pattern of correlations in the information from the brain’s circuits, we can now diagnose the type of psychiatric disorders suffered by the patient (Figure 1).
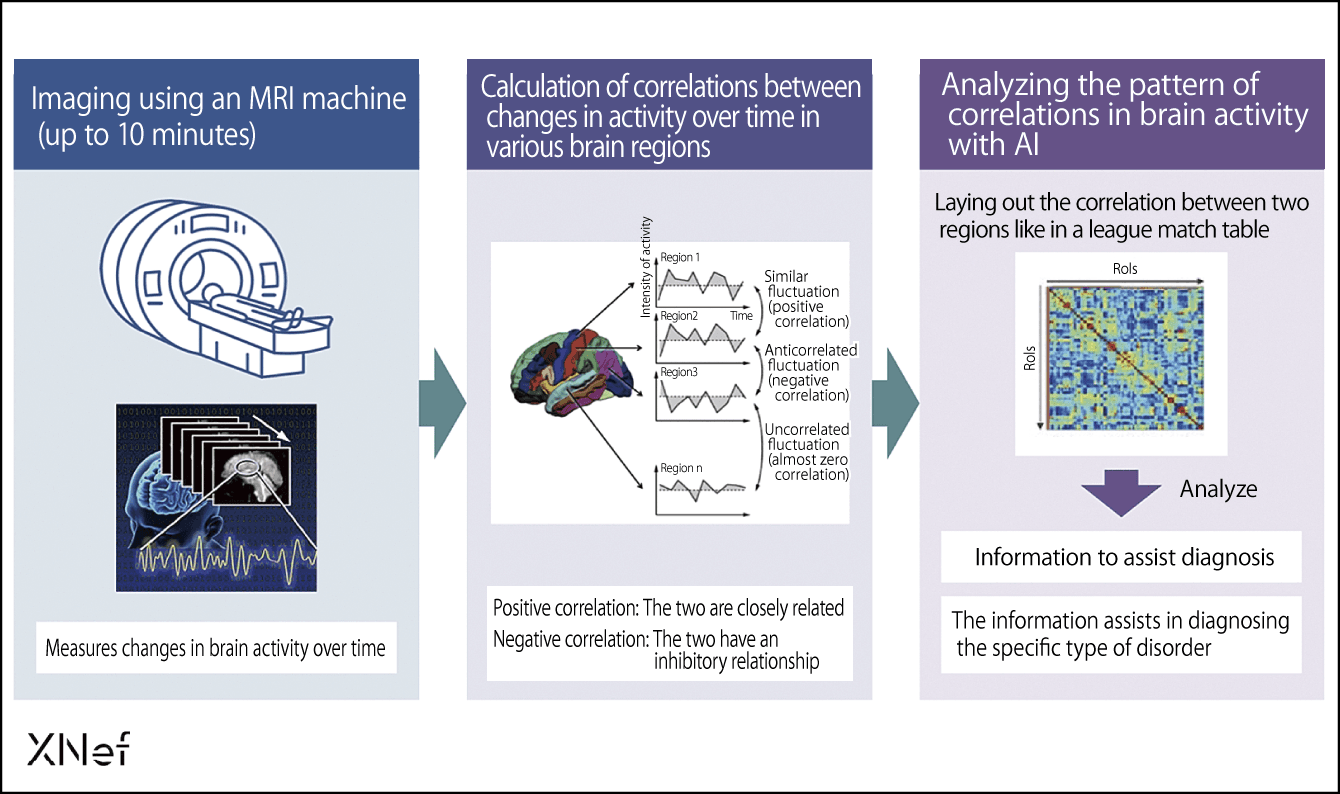
Figure 1. Information to aid diagnosis using resting-state brain functional connectivity rs-fcMRI neural circuit markersThe brain is divided into 300 regions and a conventional MRI is used to measure brain activity so that correlations between activity in each area can be calculated. When this data on patterns of correlation is analyzed with AI, we can use it as an aid to diagnose the type of psychiatric condition.
We decided to try combining AI, neuroscience, and imaging to establish biomarkers (indicators based on substances in the body) as a diagnostic aid, in a similar way to those used in other clinical fields. A single psychiatric disorder contains various types of the disorder that differ in bioscientific terms. “Depression” and “schizophrenia” are diagnoses, but not the actual disease names.
Despite this, selective serotonin reuptake inhibitors (SSRIs) are currently used for many psychiatric disorders. SSRIs were once called “wonder drugs,” but they are effective on only around 40% of patients with depression. Nevertheless, physicians tend to prescribe them for various psychiatric disorders, with the attitude, “Let’s try SSRIs first.”
If biomarkers can be established, it will be possible to link the most appropriate diagnosis with the most appropriate therapy in objective terms, even when two patients have the same psychiatric disorder. In other words, a physician will be able to state, “This patient has this type, so this type of drug will be best for them, while cognitive behavioral therapy would be better for that person and repetitive transcranial magnetic stimulation (rTMS) would suit this other person.” This kind of medical care is known by such terms as precision medicine and individualized medicine.
Being able to distinguish between different types of psychiatric disorder based on neural circuits will also lead to technologies that support drug discovery.
To develop biomarkers, we had data from 3,000 samples of neural circuits taken from a group of people with major depressive disorder (MDD) and a group of healthy individuals (healthy control, HC) at 13 facilities across the country, including Hiroshima University, Kyoto University, the University of Tokyo, and Showa University. We used this data to compile an AI program capable of distinguishing between people with MDD and HC.
With the aid of this program, data can be divided into distribution curves for patients with MDD (red) and for HC (blue); a neural circuit weighting higher than the threshold value for the classification algorithm can be judged to indicate MDD, while a lower one indicates HC (Figure 2). Alternatively, the information can be provided in the form of a percentage probability of MDD.
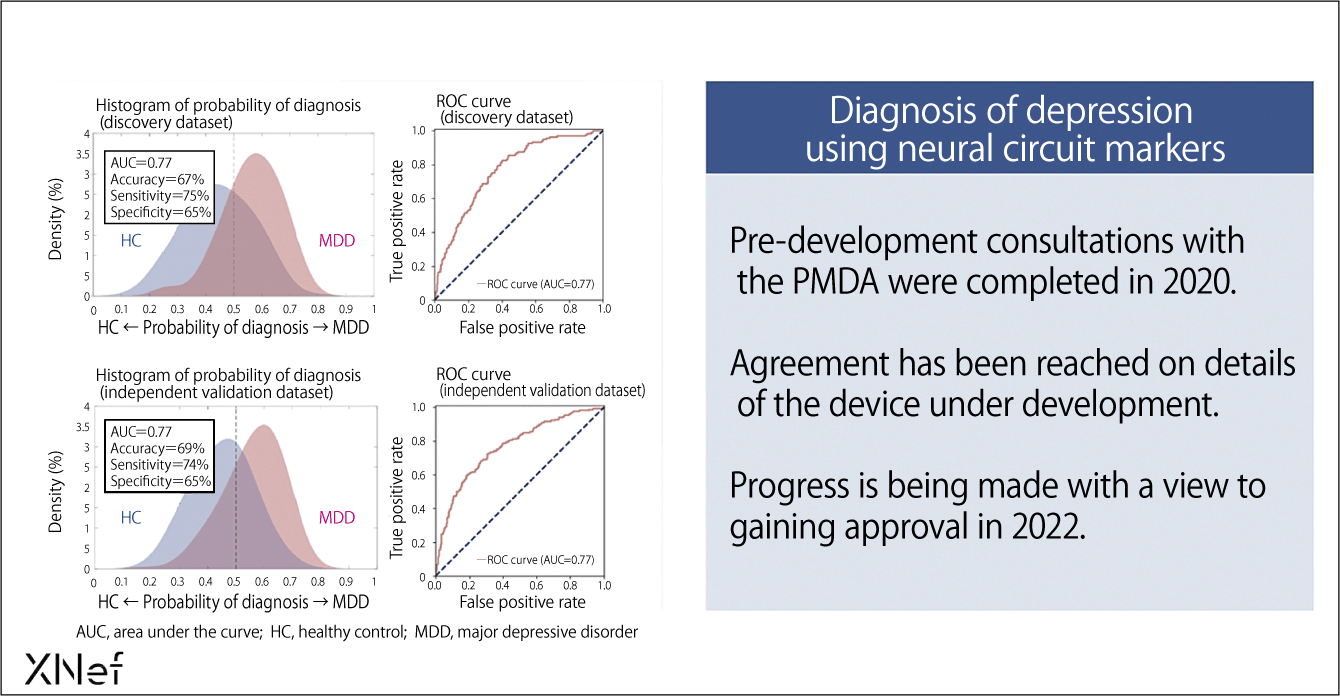
Figure 2. Information from neural circuits to aid diagnosis also improves the conformity of diagnosis in ordinary medicineThe patient’s neural circuit information is analyzed and, if it falls to the right of the threshold value (dotted line) on the distribution map, there is a high probability of their having depression, whereas the probability is low if it falls to the left of the threshold. The horizontal axis below indicates the probability of having depression, so subtracting that probability from 1 indicates the probability that a person is not depressed.
This research leads to an extension of neurofeedback, a method of visualizing and training brain activity. While looking at the activity status of the brain of a patient with a psychiatric disorder visualized using fMRI, a physician will theoretically be able to say things like, “This region has positive connectivity, but we might be able to treat your disease by changing it to negative connectivity.”
A new diagnostic technology based on fMRI imaging
We have developed a method called decoded neurofeedback (DecNef) that enables the brain to be guided toward a more desirable state (Figure 3). Patients with depression, schizophrenia, autism, and PTSD have various symptoms, but when DecNef was used, improvements were observed after a week, with further improvements seen another two months later. We are now about to begin a double-blind clinical trial of the technology.
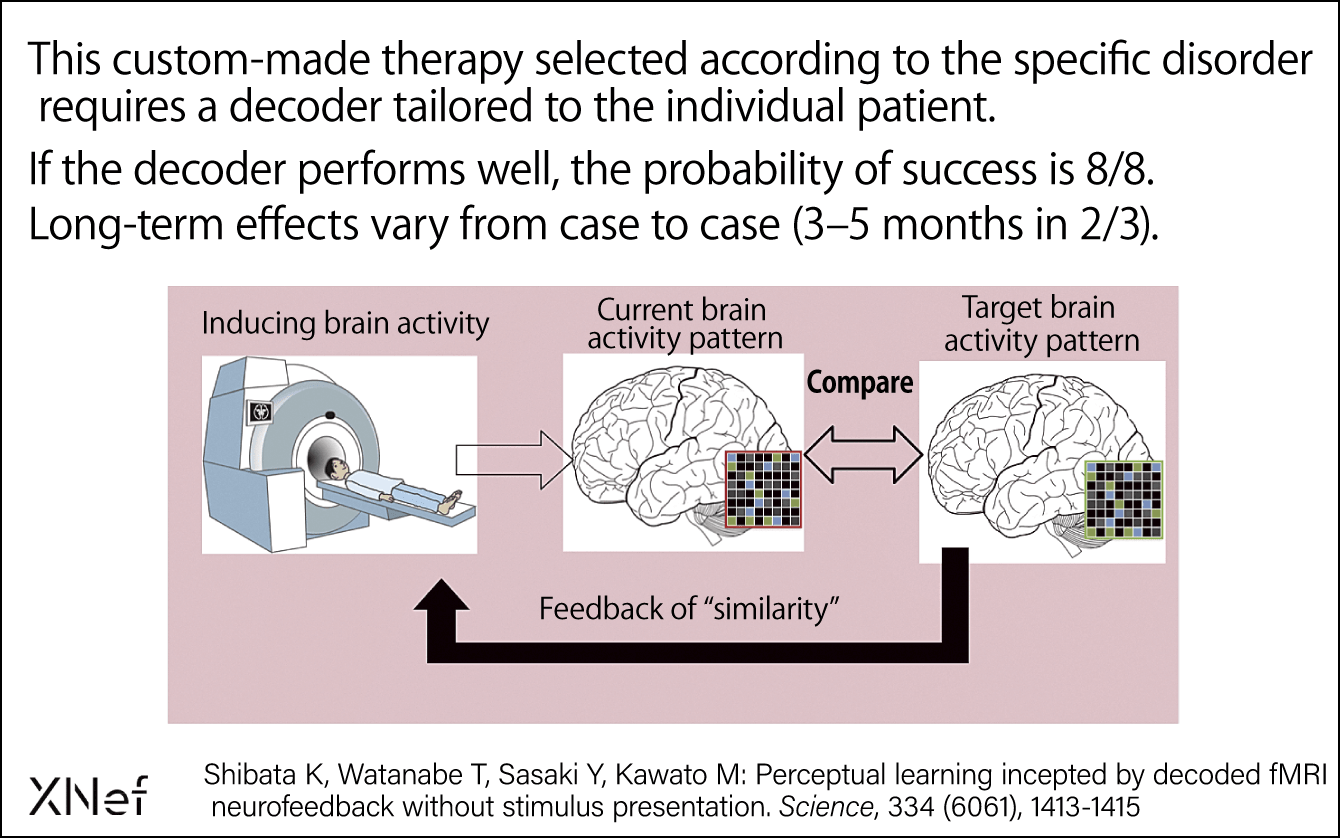
Figure 3. DecNef: Targets phobias, PTSD, obsessive-compulsive disorder (OCD), and painThe DecNef therapy for psychiatric disorders involves visualizing the patient’s brain activity pattern and providing them with the guidance needed to achieve the target brain activity pattern. It has a high therapeutic effect on phobias and PTSD, among others.
My research into the use of neuroscience in medical care began in 2008 with the brain-machine interface (BMI). I have coordinated a number of government-funded research projects focused on the use of BMI in such areas as using brain activity to move robots, enabling people who have lost the use of their limbs due to head injuries to move artificial arms and legs, and developing artificial retinas for people with vision disorders.
In conducting these studies, I ventured into the realm of using data from investigating brain activity to divide patients with psychiatric disorders into various types and even to choose treatment options. I wanted to give something back to society in the three key areas of new diagnostic technology based on fMRI imaging, drug discovery support technology, and personalized treatment techniques based on diagnosis (Figure 4), with a particular focus on the fruits of my long years of research into the first of these. Four years ago, I established the company XNef, of which I serve as CEO, while retaining my post at ATR. We at XNef aim to provide medical institutions with the Brainalyzer, a symptom-based diagnostic aid technology that uses fMRI.
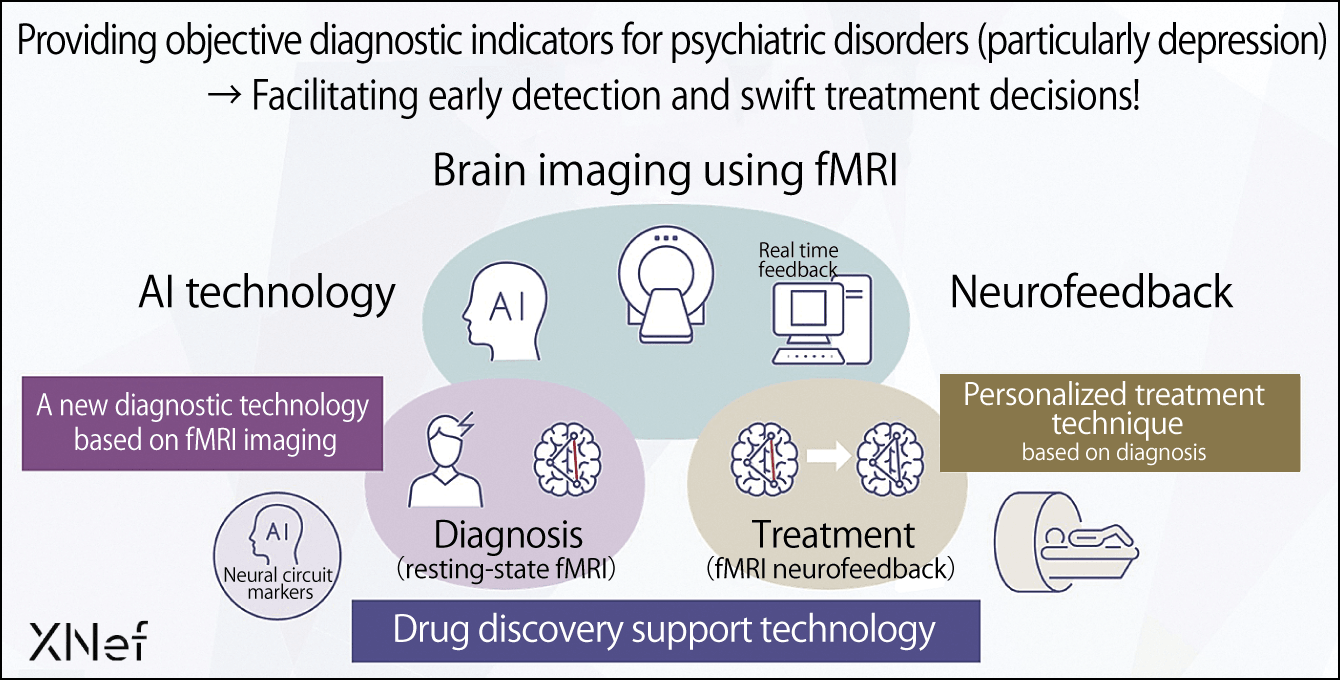
Figure 4. Vision for R&D and feeding back the results to societyBrain imaging (visualizing brain information) using fMRI will improve accuracy in diagnosing psychiatric disorders (particularly depression) and facilitate personalized treatment based on the diagnosis, as well as supporting drug discovery.
Medical institutions alter the sequence of their existing MRI machines to use them as fMRI for measuring the data of patients with psychiatric disorders and send the data to XNef. We then analyze it with our image processing server and return the results to the medical institution in question. The attending physician can use the data as one indicator for a diagnosis.
The Brainalyzer is already in practical use, and we have been in consultations with the Pharmaceuticals and Medical Devices Agency (PMDA) for the last four years regarding the device. Development is due to be completed in 2021, and we expect to receive approval as a medical device program in FY2022.
I have a dream that, by five years from now, we will be able to divide MDD into different types by looking at brain circuits. In addition, I want to work toward a situation in which, within the next 10 years, we will be able to expand the range of conditions capable of being divided by type from MDD to all developmental and psychiatric disorders such as schizophrenia and autism, and have options for treating them by means of neurofeedback.